Top 10 Mistakes In Running A Growth Team
I’ve been working in Growth for 10 years now and I wanted to reflect on the top 10 mistakes I’ve seen teams make from either teams I worked on directly or mistakes I’ve seen from talking to Growth teams at other startups over the past 10 years. For each lesson I also linked to an applicable blog post that goes into detail if you’re interested in finding out more. So, without further ado, here are the top 10 mistakes I’ve seen in Growth.
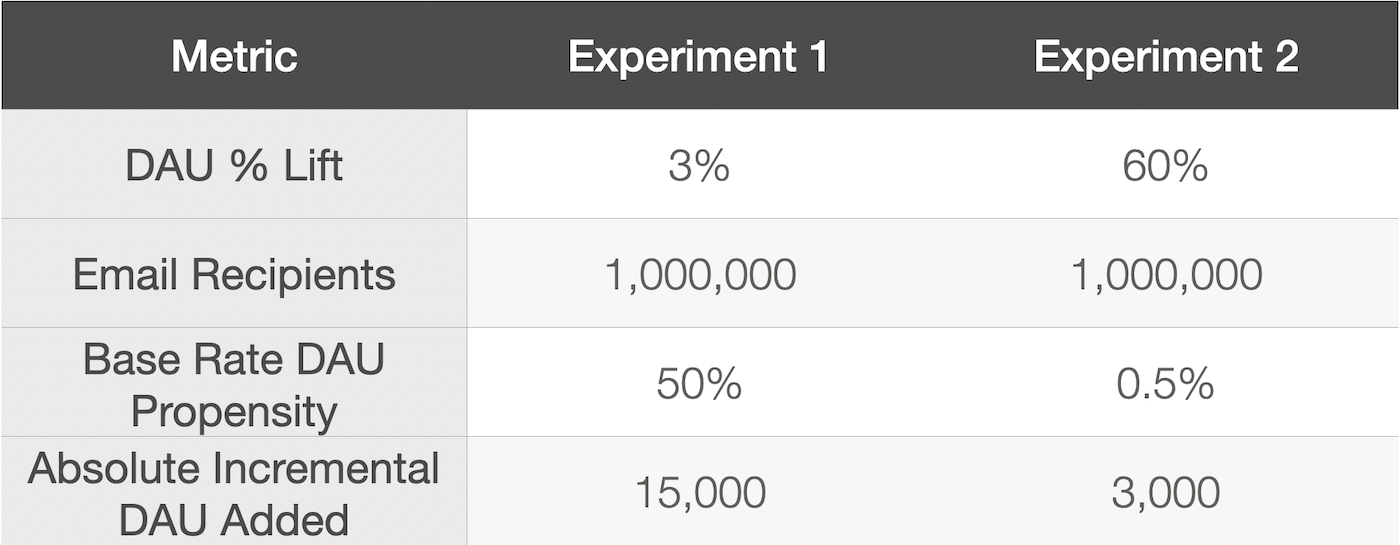
1) Using Percentage Gains
The first mistake I see for teams new to Growth is looking at all experiment results in terms of percentage gains instead of absolute number of incremental users taking an action. Percentage gains are meaningless because they are heavily influenced by the base rate for the audience in the experiment, but each experiment has different sets of users that have a different base rate. For example, if you send an email to a set of low engaged users and increase DAU amongst that group by 100% and send a different email to a set of highly engaged users and increase DAU by 5%, it is hard to tell which experiment was actually more impactful because the base rate for the propensity to be active is very different for those two groups. Experiment impact is the currency of Growth, so you should always use the absolute number of incremental users added for each experiment rather than percentage gains.
Read More: The Wrong Way to Analyze Experiments
2) Goaling On The Wrong Metric
Mistake #2 is goaling on metrics that are not tied to long term business success. A classic example is goaling a team on signups. Driving a ton of signups without retaining any of the users might make topline active user numbers look good for a bit, but they will eventually collapse when many of those users churn. You want to goal and measure on a metric that is indicative of users getting value from the product and long-term success of the business. However, be aware that if you choose an action that is too far down funnel, you may find that you are often unable to get statistical significance on any of your experiments. When picking a metric, you have to strike the right balance between a metric that indicates the user is getting value from the product, but is not so far down funnel you run into issues with statistical significance.
Read More: Autopsy of a Failed Growth Hack
3) Not Understanding Survivorship Bias
Survivorship Bias is a logical error where you draw insights based on a population but fail to account for the selection process that created that population. This can show up in a number of ways in Growth. A few examples:
- Your existing active userbase is a biased population since they got enough value from the product to stick around, while those who did not get enough value churned. This bias can impact experiment results when making major product changes such as introducing a major new feature.
- For products at scale, conversion rates can decay over time. As more people see a conversion prompt and convert, you’re left with an increasing percentage of the population who have already seen the conversion prompt multiple times and did not find it compelling enough to convert.
- Your email program has a big survivorship bias because people who don’t like your emails quickly unsubscribe. This can impact experiments when making big changes to content or frequency. You may not see what the true long-term impact of that change would be unless you zero in on how it impacts brand new users.
The way to overcome survivorship bias is to figure out if there is a way to identify a less biased population (ex: new users) and make sure to look at that segment of users when analyzing the experiment.
Read More: One Simple Logic Error That Can Undermine Your Growth Strategy
4) Not Looking At User Segments Critical To Future Growth
One major lever for growing your userbase is expanding your product market fit to users that aren’t big users of the product today. Examples could be expanding the product to new industries, verticals, demographics, counties, etc. However, your existing core audience will dominate experiment results since they make up the majority of the userbase today. When going after new audiences, it is important to look at not just the overall experiment results, but also segmenting the experiment results and looking at how it impacted audiences you are trying to expand into. You should do this for all experiments, not just the experiments targeted towards that segment. Otherwise, you may keep shipping things that are good for your core audience but may not be a fit for audiences where you hope your future growth will come from.
Read More: The Adjacent User Theory
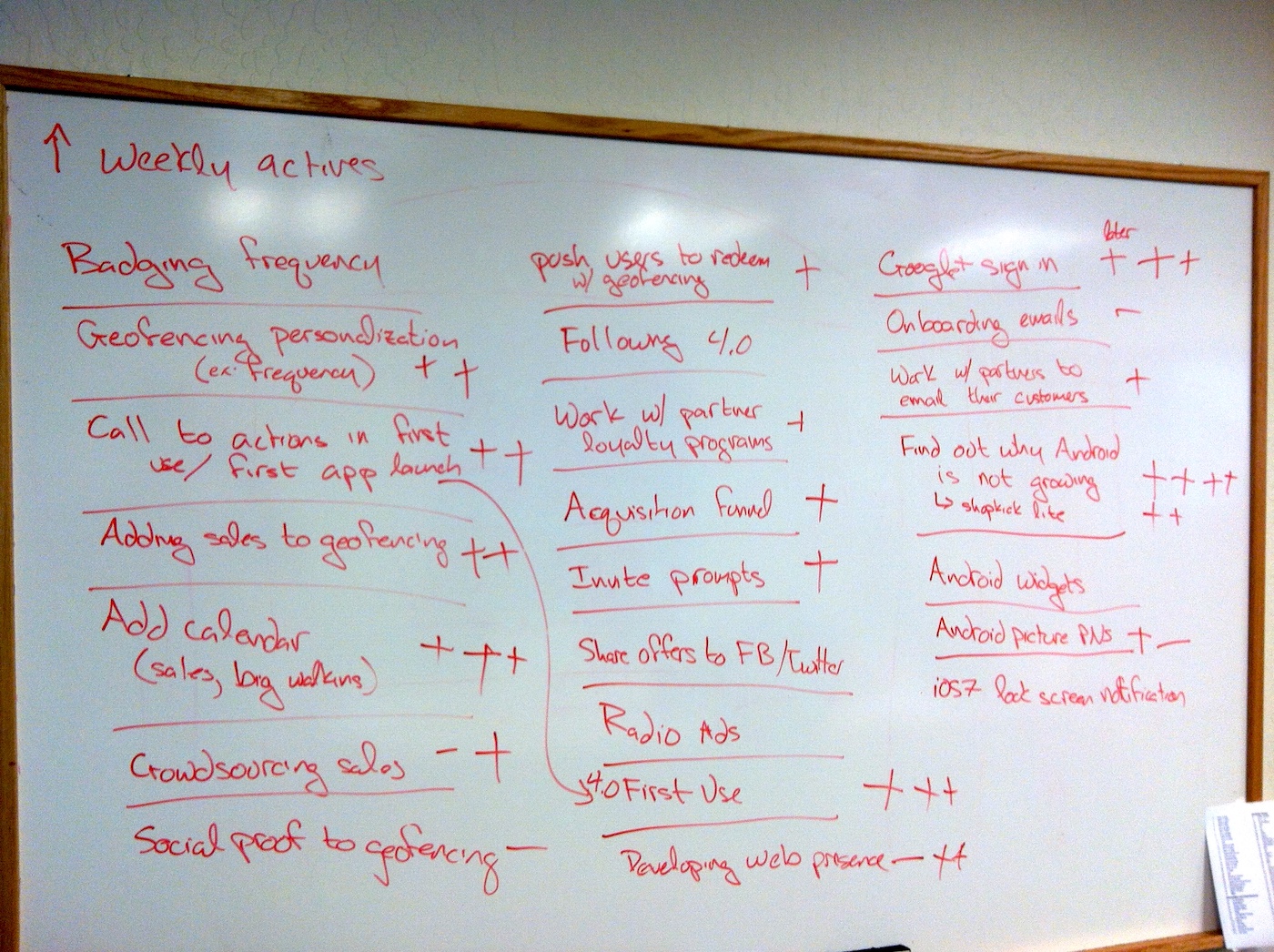
5) Not Having a Rigorous Process for Setting Your Roadmap
On the first Growth team I worked on, the way we decided on our roadmap was by brainstorming a bunch of ideas on a whiteboard and then having everyone vote on their favorite ideas. The ideas with the most votes made it into our roadmap and what we ultimately worked on for the quarter. After using this process for a couple quarters, we started to realize that a lot of the experiments coming out of this process were not generating a ton of impact. Introspecting on that process we realized some of the deficiencies with that approach were 1) ideas were not well researched and just based on what people could think of off the top of their heads, and 2) votes were made in the absence of data and were based on gut feeling which was often wrong. I’ve become a huge believer that the single biggest lever for a Growth team to increase its impact is to improve their process around how they come up with experiment ideas and how they decide which experiment idea to work on. At Pinterest we implemented a process we called Experiment Idea Review where every member of the team comes up with an idea, takes time to research it and dig into the data, then pitches it to the team and the then has a discussion to decide if we should pursue it.
Read More:
How Pinterest Supercharged Its Growth Team With Experiment Idea Review
Managing Your Growth Team’s Portfolio: A Step-by-step Guide
6) Not Staffing Teams Correctly
There are three ways I see Growth teams incorrectly staffed:
- Startups trying to start an initial Growth team with just 1 or 2 engineers. For early-stage startups (e.g. <10 employees), Growth should be everyone’s job. When you get to the point though of forming a dedicated Growth team, you need a minimum of 3 engineers to start the team. With any less than that, the team cannot move quickly enough because they are stretched too thin.
- Staffing teams with engineers that are not going to be retained on the team long term. Growth often involves a lot of iterative experimentation. Generally, the engineers that often do best in Growth are often motivated by driving business impact, are full-stack and capable of working across different platforms and technologies. Engineers that are primarily motivated by building big new features or solving big technical challenges (although Growth does have some big technical challenges!) tend not to be retained in Growth because they don’t find the work in Growth motivating.
- Not having the right skill sets in the team. This can come in two flavors: overreliance on other teams or just not having the right level of expertise. Moving quickly and having a high velocity of experimentation and iteration is critical to a Growth team’s success. For instance, if the company has a centralized iOS team that implements everything on iOS and the Growth team needs to constantly request time on that team’s roadmap to implement things instead of being able to do it themselves, the reliance on the iOS team will really slow the Growth team down and create a lot of friction in getting things done because different teams have different goals and priorities which then need to be negotiated to get on the roadmap. The other flavor is not having the right level of expertise. If a team is faced with a problem set that is best solved through machine learning and they don’t have machine learning experts on the team, the team may try to solve the problem using non-ML approaches which are not as impactful or attempt to tackle the problem using ML, but not have the expertise to successfully deliver an impactful solution.
Read More:
3 Habits of a Highly Effective Growth Team
Hiring Growth Engineers Is Not Impossible
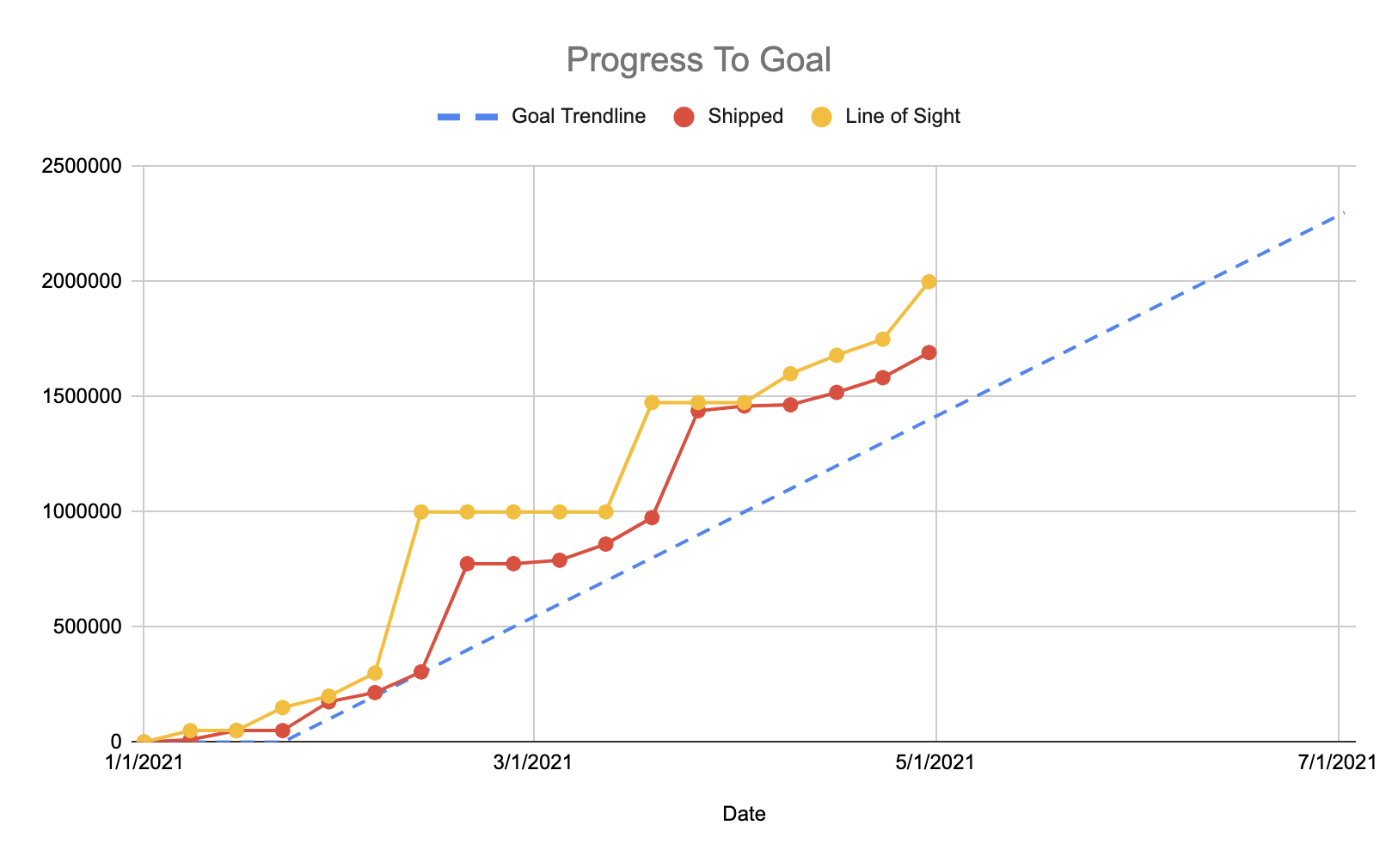
7) Losing Sight Of The Goal
Growth teams generally have a metric goal for the quarter. In some of the early Growth teams I was on, as we executed on the quarter, we were heads down and focused on the roadmap and shipping experiment on a week-to-week basis and didn’t really check in on the goal very often. In spite of this, we kept hitting goals until finally one quarter we missed our goals and missed by a large margin.
What we realized is we needed a process to regularly review how we were doing on a weekly basis so we could be nimble and make adjustments. In our team weekly meeting, we started reviewing how we were doing relative to goal. It was also important to have a trendline to help us determine how we were pacing relative to goal and help us understand if we were ahead or if we were falling behind. Having this process really enabled us to catch early on if we were falling behind goal and rally the team to figure out what we could do to get back on track.
Read More:
The Right Way To Set Growth Goals
5 Principles For Goaling Your Growth Team
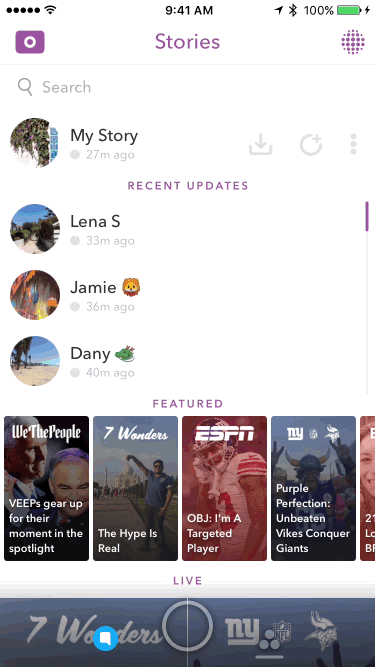
8) Building Features Hoping They Drive Growth
In enterprise software, building features often correlates with growth because it increases the number of customers whose requirements you now meet. However, I sometimes see consumer startups invest a ton of time building many features or go through big redesigns in hopes that they will drive user growth or engagement. Features can drive growth; some good examples are when Facebook added the ability to upload photos and tag your friends or when Snapchat added Stories. However I see consumer startups all too often building features because it is cool or exciting and hoping it will drive growth without understanding what makes a feature drive growth. In consumer products for a feature to drive growth, it generally must meet the following criteria:
A) The feature has to impact the majority of the userbase (i.e. it can’t be for a niche set of users, except in the scenario where building the feature for that niche set will then have a much bigger impact on the rest of the userbase).
B) The feature creates an engagement loop. Typically, the engagement loop is created by notifying users on a regular basis. The content in those emails and notifications in that engagement loop needs to be compelling enough that it maintains a high engagement rate over time.
C) The feature is a step-change improvement in the core value of the product for a majority of the userbase.
Taking Snapchat Stories for example, it is clear how it fit these three criteria:
A) Stories was available to the entire userbase and I suspect the vast majority of their active users posts to their story or views the stories of others.
B) Stories disappeared after 24 hours which created an engagement loop by getting users to build an internal trigger to check the app regularly to view the stories of their friends or risk missing out on seeing what their friends were up to. For less engaged users, Snapchat would notify them when a friend posted a story to bring them back.
C) Stories were a big increase in product value for Snapchat. Instead of just being a direct messaging product to send or receive messages from close friends, Stories significantly increased the amount of engaging content available to users.
Read More: When Do Features Drive Growth?
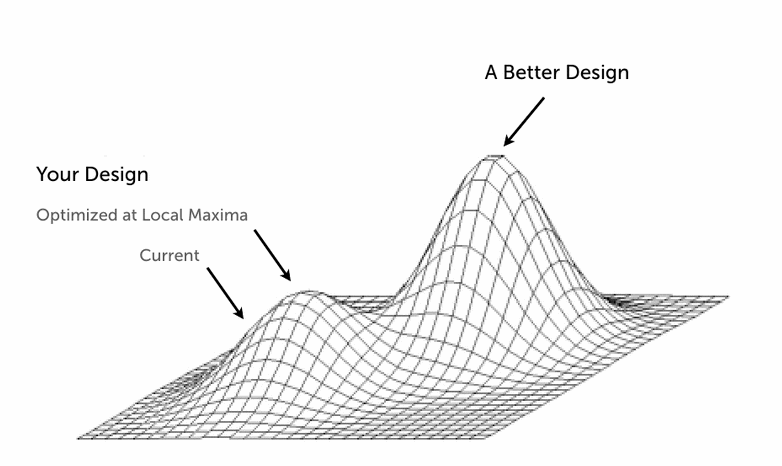
9) Getting Stuck In A Local Maximum
Growth involves a lot of iteration and optimization. That can sometimes lead to optimizing yourself into a corner, where there may be a better performing experience out there, but you cannot iterate your way to it because every step iteration from your current experience will be negative.
To get out of this local maximum you need to make a bet and try a few divergent experiences. It is unlikely any of those divergent experiences will beat your control off the bat though, since you have spent months or years optimizing your current control experience. You need to be willing to invest in multiple iterations on those divergent experiences until they start to outperform control.
Read More: How To Get Over Inevitable Local Maximum?
10) Big Bets Without Clear Milestones
That brings us to our last mistake, which is making big bets without clear milestones. I’ve seen times when teams have executed on big bets without a plan or regular checkpoints to decide if the bet is worth continuing. I’ve also seen times when a team takes on a big bet with a mentality that it will ship at all costs and failure is not an option. In either of these two modes, if the bet doesn’t pan out as expected, the team can then be stuck in a tough situation where they have invested a large sunk cost, things aren’t making progress, but the team keeps plodding along trying to get the bet to work. This can come at the cost of not investing those resources elsewhere into something that is working or at the cost of not taking a step back and pursuing a different approach. When taking on a big bet, it is crucial to set up a regular cadence for reviewing the status of the bet, making a go/no go decision on if the team should continue to invest in the bet, and setting out a clear plan and milestones upfront to help inform those checkpoints and go/no go decisions.
Read More:
Managing Your Growth Team’s Portfolio: A Step-by-Step Guide
I hope that after reading these 10 mistakes and lessons you can learn from them and can avoid some of the biggest pitfalls I’ve seen in the past 10 years in Growth.